Billions of dollars have flooded into GenAI, attracting major tech players to look into its potential beyond mere trivia such as image/video processing and knowledge summaries. Yet, its integration into industrial settings encounters notable hurdles, particularly the "hallucination" issue. Utilizing GenAI for problem-solving demands a profound understanding of the specific issue at hand.
Present GenAI endeavors predominantly center on tasks like knowledge modeling but often falter in comprehending the complexities of problems, resulting in hallucinations or irrelevant outputs.
Now, with discussions pivoting towards actual deployments in industrial contexts, stakeholders find themselves grappling with how to attain "return on investment" while pouring further billions into GenAI. So, before we proceed, let's see how we arrived at this stage.
Throughout my engineering career, I've been immersed in challenges related to ML/AI, automation, troubleshooting, and control systems. Traditionally, troubleshooting has been viewed as a two-fold process: thoroughly understanding the problem before attempting any remedy or solution—analogous to diagnosing an illness before prescribing medication.
When I co-founded Waylay a decade ago, my vision was to introduce explainable automation, facilitated by a Low Code environment, as the quintessential IoT application. Initially, I presumed this vision would be self-evident, requiring little explanation. However, I soon encountered two distinct challenges with this message.
Firstly, business and sales professionals understandably seek to streamline the deployment cycle for new applications, thus are averse to the notion of requiring an expert for issue resolution. Our tools were perceived as expert systems, where knowledge workers would articulate their intent using our platform. Consequently, alternatives such as unsupervised anomaly detection, promising a magic solution without mentioning "training nuances", appeared more appealing on the surface. Despite the fact that Waylay also incorporates ML capabilities, our primary message inadvertently conveyed otherwise.
Secondly, the notion of being a low code automation tool was misconstrued. For sales personnel, "low code" connoted minimal or zero coding involvement—what became known as the "citizen development" narrative. Despite my efforts through blogs and posts to rectify this perception, it remained largely unchanged.
Returning to the genesis of this blog, we're confronted with anomaly detection-like approaches devoid of issue clarification and GenAI models prone to erroneous outcomes.
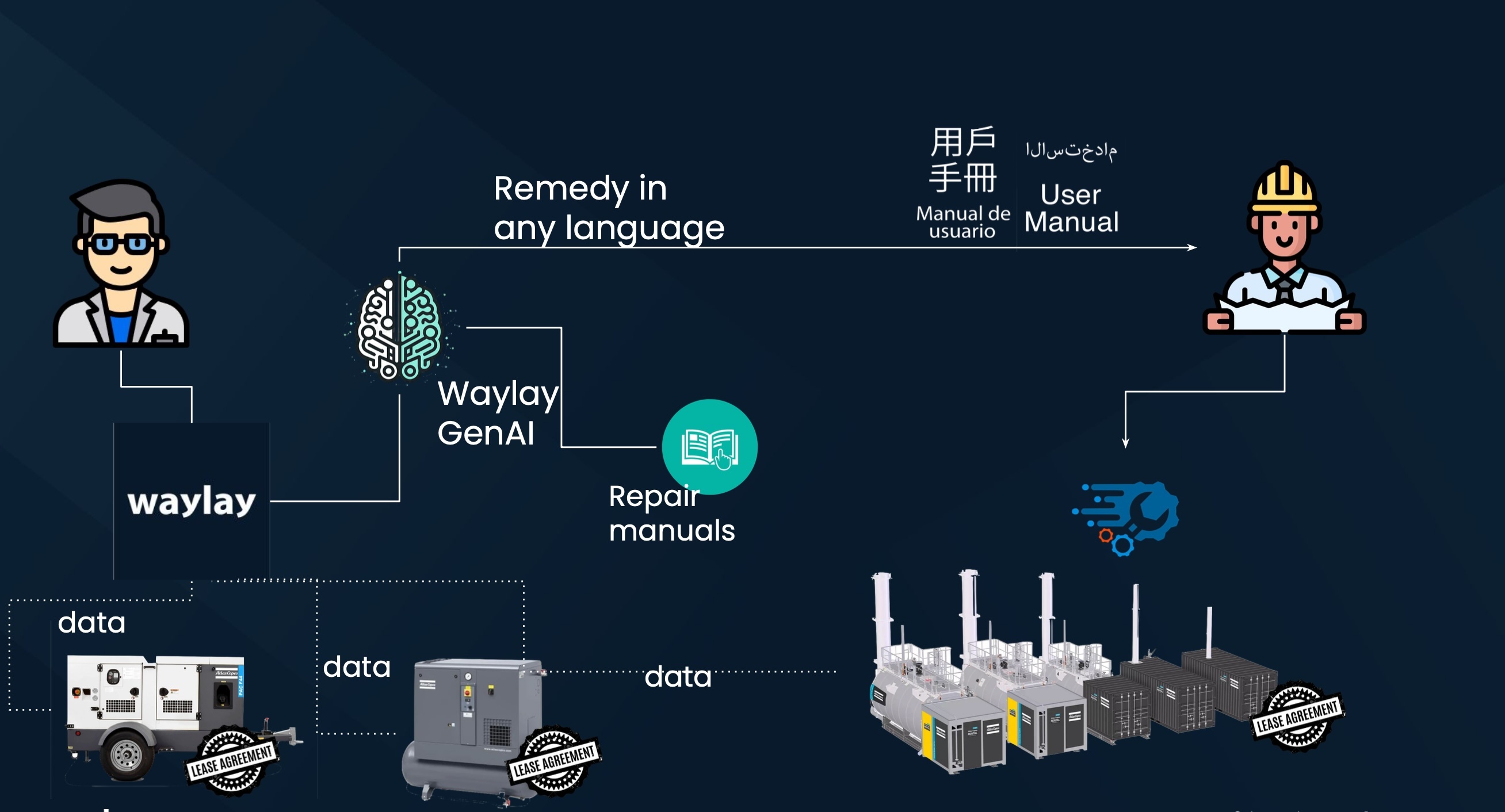
Waylay's innovative approach confronts these challenges head-on. By integrating machine data, causal graphs, and outcome insights into the training process, Waylay not only enhances the efficacy of GenAI models but also ensures their high explainability, signifying a significant leap forward in AI deployment.
We combine historical records/manuals with real-time machine data to find the root cause and provide the right remedy in any language - reducing the risk of human error, ensuring consistency that allows human workers to focus on more complex and strategic aspects of their jobs.
Our initial testing with a client serves as a testament to this advancement.
If you're eager to explore further, consider watching this video for additional insights. Better yet, if you're keen on implementing these strategies within your own organization, don't hesitate to reach out to Waylay at sales@waylay.io